Catalogue Search | MBRL
نتائج البحث
MBRLSearchResults
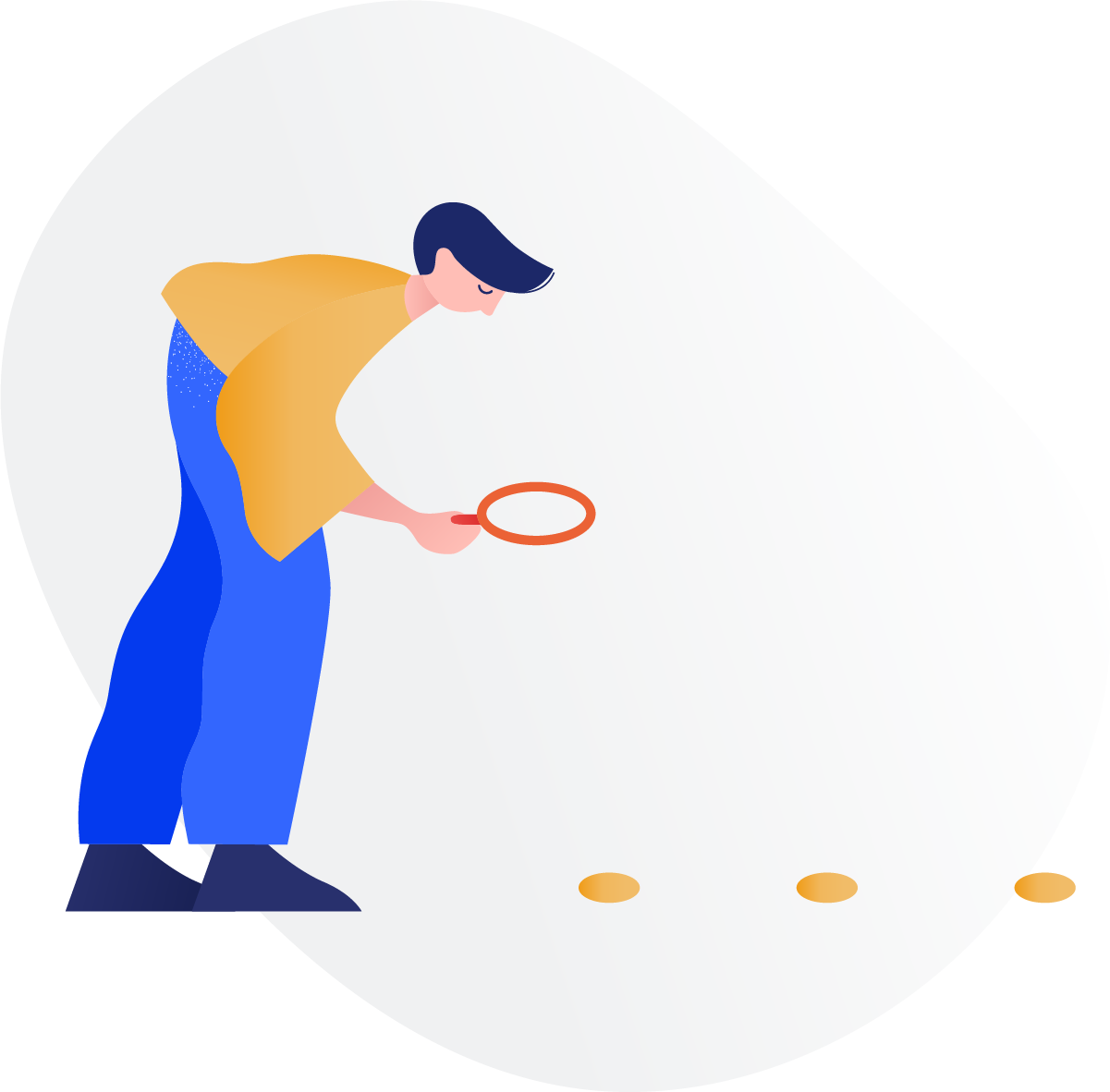
وجه الفتاة! هناك خطأ ما.
أثناء محاولة إضافة العنوان إلى الرف ، حدث خطأ ما :( يرجى إعادة المحاولة لاحقًا!
-
الضبطالضبط
-
مُحَكَّمةمُحَكَّمة
-
مستوى القراءةمستوى القراءة
-
نوع المحتوىنوع المحتوى
-
السنةمن:-إلى:
-
المزيد من المرشحاتالمزيد من المرشحاتنوع العنصرلديه النص الكاملالموضوعالناشرالمصدرالمُهدياللغةمكان النشرالمؤلفينالموقع
منجز
مرشحات
إعادة تعيين
133
نتائج ل
"So, Yan-kit"
صنف حسب:
My Top Five Chinese Foods
بواسطة
YAN-KIT SO
1995
1: Fermented black beans Soya beans are fermented, preserved in salt and ginger then dried. To make the versatile black bean sauce used all over China, they are then mashed with a little water or, better still, Chinese wine, and then combined with chopped garlic and vegetable oil. For mussels in black bean sauce, first stir-fry the mashed black beans and chopped garlic in a wok, add the mussels, toss to coat them with the sauce, then cover for a minute until the mussels open.
Newspaper Article
ENSO Transition, Duration, and Amplitude Asymmetries
بواسطة
Vecchi, Gabriel A.
,
Choi, Kit-Yan
,
Wittenberg, Andrew T.
في
Asymmetry
,
Climate
,
Climate change
2013
The El Niño–Southern Oscillation (ENSO) exhibits well-known asymmetries: 1) warm events are stronger than cold events, 2) strong warm events are more likely to be followed by cold events than vice versa, and 3) cold events are more persistent than warm events. Coupled GCM simulations, however, continue to underestimate many of these observed features.
To shed light on these asymmetries, the authors begin with a widely used delayed-oscillator conceptual model for ENSO and modify it so that wind stress anomalies depend more strongly on SST anomalies (SSTAs) during warm conditions, as is observed. Then the impact of this nonlinearity on ENSO is explored for three dynamical regimes: self-sustained oscillations, stochastically driven oscillations, and self-sustained oscillations disrupted by stochastic forcings. In all three regimes, the nonlinear air–sea coupling preferentially strengthens the feedbacks (both positive and delayed negative) during the ENSO warm phase—producing El Niños that grow to a larger amplitude and overshoot more rapidly and consistently into the opposite phase, than do the La Niñas. Finally, the modified oscillator is applied to observational records and to control simulations from two global coupled ocean–atmosphere–land–ice models [Geophysical Fluid Dynamics Laboratory Climate Model version 2.1 (GFDL CM2.1) and version 2.5 (GFDL CM2.5)] to elucidate the causes of their differing asymmetries.
Journal Article
Twitter mining for ontology-based domain discovery incorporating machine learning
بواسطة
Yan Kit, Chan
,
Abu-Salih, Bilal
,
Wongthongtham, Pornpit
في
Algorithms
,
Artificial intelligence
,
Business intelligence
2018
Purpose
This paper aims to obtain the domain of the textual content generated by users of online social network (OSN) platforms. Understanding a users’ domain (s) of interest is a significant step towards addressing their domain-based trustworthiness through an accurate understanding of their content in their OSNs.
Design/methodology/approach
This study uses a Twitter mining approach for domain-based classification of users and their textual content. The proposed approach incorporates machine learning modules. The approach comprises two analysis phases: the time-aware semantic analysis of users’ historical content incorporating five commonly used machine learning classifiers. This framework classifies users into two main categories: politics-related and non-politics-related categories. In the second stage, the likelihood predictions obtained in the first phase will be used to predict the domain of future users’ tweets.
Findings
Experiments have been conducted to validate the mechanism proposed in the study framework, further supported by the excellent performance of the harnessed evaluation metrics. The experiments conducted verify the applicability of the framework to an effective domain-based classification for Twitter users and their content, as evident in the outstanding results of several performance evaluation metrics.
Research limitations/implications
This study is limited to an on/off domain classification for content of OSNs. Hence, we have selected a politics domain because of Twitter’s popularity as an opulent source of political deliberations. Such data abundance facilitates data aggregation and improves the results of the data analysis. Furthermore, the currently implemented machine learning approaches assume that uncertainty and incompleteness do not affect the accuracy of the Twitter classification. In fact, data uncertainty and incompleteness may exist. In the future, the authors will formulate the data uncertainty and incompleteness into fuzzy numbers which can be used to address imprecise, uncertain and vague data.
Practical implications
This study proposes a practical framework comprising significant implications for a variety of business-related applications, such as the voice of customer/voice of market, recommendation systems, the discovery of domain-based influencers and opinion mining through tracking and simulation. In particular, the factual grasp of the domains of interest extracted at the user level or post level enhances the customer-to-business engagement. This contributes to an accurate analysis of customer reviews and opinions to improve brand loyalty, customer service, etc.
Originality/value
This paper fills a gap in the existing literature by presenting a consolidated framework for Twitter mining that aims to uncover the deficiency of the current state-of-the-art approaches to topic distillation and domain discovery. The overall approach is promising in the fortification of Twitter mining towards a better understanding of users’ domains of interest.
Journal Article
Relational Learning Analysis of Social Politics using Knowledge Graph Embedding
بواسطة
Chan, Kit Yan
,
Beheshti, Amin
,
Aljarah, Ibrahim
في
Application Papers
,
Artificial Intelligence
,
Chemistry and Earth Sciences
2021
Knowledge Graphs (KGs) have gained considerable attention recently from both academia and industry. In fact, incorporating graph technology and the copious of various graph datasets have led the research community to build sophisticated graph analytics tools, which has extended the application of KGs to tackle a plethora of real-life problems in dissimilar domains. Despite the abundance of the currently proliferated generic KGs, there is a vital need to construct domain-specific KGs. Further, quality and credibility should be assimilated in the process of constructing and augmenting KGs, particularly those propagated from mixed-quality resources such as social media data. For example, the amount of the political discourses in social media is overwhelming yet can be hijacked and misused by spammers to spread misinformation and false news. This paper presents a novel credibility domain-based KG Embedding framework. This framework involves capturing a fusion of data related to politics domain and obtained from heterogeneous resources into a formal KG representation depicted by a politics domain ontology. The proposed approach makes use of various knowledge-based repositories to enrich the semantics of the textual contents, thereby facilitating the interoperability of information. The proposed framework also embodies a domain-based social credibility module to ensure data quality and trustworthiness. The utility of the proposed framework is verified by means of experiments conducted on two constructed KGs. The KGs are then embedded in low-dimensional semantically-continuous space using several embedding techniques. The effectiveness of embedding techniques and social credibility module is further demonstrated and substantiated on link prediction, clustering, and visualisation tasks.
Journal Article
Nonlinear Zonal Wind Response to ENSO in the CMIP5 Models
بواسطة
Vecchi, Gabriel A.
,
Choi, Kit-Yan
,
Wittenberg, Andrew T.
في
Atmospheric circulation
,
Atmospheric models
,
Brackish
2015
The observed equatorial Pacific zonal wind response during El Niño tends to be stronger than during La Niña. Most global coupled climate models in phase 5 of CMIP (CMIP5) exhibit such nonlinearity, although weaker than observed. The wind response nonlinearity can be reproduced by driving a linear shallow water atmospheric model with a model’s or the observed precipitation anomalies, which can be decomposed into two main components: the zonal and meridional redistribution of the climatological precipitation. Both redistributions contribute comparably to the total rainfall anomalies, whereas the zonal redistribution plays the dominant role in the zonal wind response. The meridional redistribution component plays an indirect role in the nonlinear wind response by limiting the zonal redistribution during La Niña and thus enhancing the nonlinearity in the wind response significantly. During La Niña, the poleward movement of the ITCZ/SPCZ reduces the equatorial zonal-mean precipitation available for the zonal redistribution and its resulting zonal wind response. Conversely, during El Niño, the equatorward movement of the ITCZ and SPCZ do not limit the zonal redistribution of precipitation. The linear equatorial zonal wind response to ENSO is found to have a significant linear correlation with the equatorial central Pacific climatological precipitation and SST among the CMIP5 models. However, no linear correlation is found between the nonlinear equatorial zonal wind response and the climatological precipitation.
Journal Article
Time-aware domain-based social influence prediction
بواسطة
Chan, Kit Yan
,
Bremie, Bushra
,
Issa, Tomayess
في
Communications Engineering
,
Computational Science and Engineering
,
Computer Science
2020
Online social networks have established virtual platforms enabling people to express their opinions, interests and thoughts in a variety of contexts and domains, allowing legitimate users as well as spammers and other untrustworthy users to publish and spread their content. Hence, it is vital to have an accurate understanding of the contextual content of social users, thus establishing grounds for measuring their social influence accordingly. In particular, there is the need for a better understanding of domain-based social trust to improve and expand the analysis process and determining the credibility of Social Big Data. The aim of this paper is to determine domain-based social influencers by means of a framework that incorporates semantic analysis and machine learning modules to measure and predict users’ credibility in numerous domains at different time periods. The evaluation of the experiment conducted herein validates the applicability of semantic analysis and machine learning techniques in detecting highly trustworthy domain-based influencers.
Journal Article